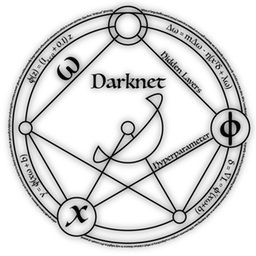
Darknet - Convolutional Layer
All GPU implementations have been ignored
Written by ivanpp for fun, contact me: [email protected]
Initialize a convolutional layer
make_convolutional_layer
convolutional_layer make_convolutional_layer(int batch, int h, int w, int c, int n, int groups, int size, int stride, int padding, ACTIVATION activation, int batch_normalize, int binary, int xnor, int adam)
{
int i;
// create a convolutional_layer(layer) type variable l, initialize all struct members to 0.
convolutional_layer l = {0};
l.type = CONVOLUTIONAL;
// Get the params
l.groups = groups; // optional: weight sharing across 'groups' channels
l.h = h; // input height
l.w = w; // input width
l.c = c; // input channels
l.n = n; // num of filters
l.binary = binary; // optional: ?
l.xnor = xnor; // optional: ?
l.batch = batch; // num of image per batch
l.stride = stride; // stride of the conv operation
l.size = size; // kernel size of filters
l.pad = padding; // padding of the conv operation
l.batch_normalize = batch_normalize; // optional: bn after conv
// Allocate memory (for conv weight and conv weight_update)
l.weights = calloc(c/groups*n*size*size, sizeof(float)); // stored as (n*(c/groups)*size*size)
l.weight_updates = calloc(c/groups*n*size*size, sizeof(float));
l.biases = calloc(n, sizeof(float));
l.bias_updates = calloc(n, sizeof(float));
l.nweights = c/groups*n*size*size; // num of params for l.weights
l.nbiases = n; // num of params for l.biases
// Initialize weights to random_uniform
float scale = sqrt(2./(size*size*c/l.groups));
for(i = 0; i < l.nweights; ++i) l.weights[i] = scale*rand_normal();
// Allocate memory (for forward and backward)
int out_w = convolutional_out_width(l); // compute output width
int out_h = convolutional_out_height(l); // compute output height
l.out_h = out_h;
l.out_w = out_w;
l.out_c = n; // output channel should be num of filter, n
l.outputs = l.out_h * l.out_w * l.out_c;
l.inputs = l.w * l.h * l.c;
l.output = calloc(l.batch*l.outputs, sizeof(float)); // for conv output(forward pass)
l.delta = calloc(l.batch*l.outputs, sizeof(float)); // for prev layer's gradient(backward pass)
// Assign forward, backward and update function
l.forward = forward_convolutional_layer;
l.backward = backward_convolutional_layer;
l.update = update_convolutional_layer;
if(binary){
l.binary_weights = calloc(l.nweights, sizeof(float));
l.cweights = calloc(l.nweights, sizeof(char));
l.scales = calloc(n, sizeof(float));
}
if(xnor){
l.binary_weights = calloc(l.nweights, sizeof(float));
l.binary_input = calloc(l.inputs*l.batch, sizeof(float));
}
if(batch_normalize){
l.scales = calloc(n, sizeof(float));
l.scale_updates = calloc(n, sizeof(float));
for(i = 0; i < n; ++i){
l.scales[i] = 1;
}
l.mean = calloc(n, sizeof(float));
l.variance = calloc(n, sizeof(float));
l.mean_delta = calloc(n, sizeof(float));
l.variance_delta = calloc(n, sizeof(float));
l.rolling_mean = calloc(n, sizeof(float));
l.rolling_variance = calloc(n, sizeof(float));
l.x = calloc(l.batch*l.outputs, sizeof(float));
l.x_norm = calloc(l.batch*l.outputs, sizeof(float));
}
if(adam){
l.m = calloc(l.nweights, sizeof(float));
l.v = calloc(l.nweights, sizeof(float));
l.bias_m = calloc(n, sizeof(float));
l.scale_m = calloc(n, sizeof(float));
l.bias_v = calloc(n, sizeof(float));
l.scale_v = calloc(n, sizeof(float));
}
l.workspace_size = get_workspace_size(l);
l.activation = activation; // which activation to use
fprintf(stderr, "conv %5d %2d x%2d /%2d %4d x%4d x%4d -> %4d x%4d x%4d %5.3f BFLOPs\n", n, size, size, stride, w, h, c, l.out_w, l.out_h, l.out_c, (2.0 * l.n * l.size*l.size*l.c/l.groups * l.out_h*l.out_w)/1000000000.);
return l;
}
Describe Sth please
Optional params:
Optional params | Forward | Backward | Update | Usage | Defined in |
---|---|---|---|---|---|
l.groups | Y | Y | N | [convolutional] | |
l.binary | TODO | TODO | TODO | [convolutional] | |
l.xnor | TODO | TODO | TODO | [convolutional] | |
l.batch_normalize | Y | Y | N | Regularization | [convolutional] |
adam | Optimization Algorithm | [net] |
Batch normalization and Adam will not be covered in this blog.
forward_convolutional_layer()
Image(or image like) input *net.input (given by the net) has the size of $[batch\times c\times h\times w]$, consider it as a $(batch, c, h, w)$ matrix.
Conv filter *l.weights has the size of $[n\times \frac{c}{groups}\times\ size\times size]$, consider it as a $(n, \frac{c}{groups}, size, size)$ matrix.
Conv bias *l.biases has the size of $[n]$, namely 1 float bias for 1 filter.
Conv output *l.output has the size of $[batch\times n\times out_h\times out_w]$, consider it as a $(batch, n, out_h, out_w)$ matrix.
Conv workspace *l.workspace should have the size of $[\frac{c}{groups}\times out_h\times out_w\times size\times size]$. But the actual size of the *net.workspace(workspace for all conv/deconv/local layers in a net) should be suitable for the layer that needs the most workspace memory. All conv/deconv/local layers share the workspace of the net. So the most of the conv/deconv/local layers are only using part of the net's workspace.
Conv Operation
Use default value for all optional params:
net.adam = 0;
l.groups = 1;
l.batch_normalize = 0;
l.binary = 0;
l.xnor = 0;
We can get the minimal implementation:
// minimal implementation of conv forward
void forward_convolutional_layer_min(convolutional_layer l, network net)
{
int i;
fill_cpu(l.outputs*l.batch, 0, l.output, 1);
int m = l.n;
int k = l.size*l.size*l.c;
int n = l.out_w*l.out_h;
for(i = 0; i < l.batch; ++i){
float *a = l.weights;
float *b = net.workspace;
float *c = l.output + i*n*m;
im2col_cpu(net.input + i*l.c*l.h*l.w, l.c, l.h, l.w, l.size, l.stride, l.pad, b);
gemm(0,0,m,n,k,1,a,k,b,n,1,c,n);
}
add_bias(l.output, l.biases, l.batch, l.n, l.out_h*l.out_w);
activate_array(l.output, l.outputs*l.batch, l.activation);
}
Now *net.input remains the same, has the size of $[batch\times c\times h\times\ w]$. For one single image in current batch, it has the size of $[c\times h\times w]$. And conv filter matrix has the shape of $(n,c,size,size)$.
Instead of using for loops to do conv operations at each input location using all the filters, we use im2col and then just do matrix multiplication.
// src/im2col.c
void im2col_cpu(float* data_im,
int channels, int height, int width,
int ksize, int stride, int pad, float* data_col)
{
int c,h,w;
int height_col = (height + 2*pad - ksize) / stride + 1; // height after reconstruct
int width_col = (width + 2*pad - ksize) / stride + 1; // width after reconstruct
int channels_col = channels * ksize * ksize; // filter(channels, ksize, ksize)
for (c = 0; c < channels_col; ++c) { // flatten the filter
int w_offset = c % ksize; // from which column
int h_offset = (c / ksize) % ksize; // from which row
int c_im = c / ksize / ksize; // from which channel
for (h = 0; h < height_col; ++h) { // iterate the reconstructed img(out_h*out_w)
for (w = 0; w < width_col; ++w) {
// mapping reconstructed img to padded img(which col)
int im_row = h_offset + h * stride;
// mapping reconstructed img to padded img(which row)
int im_col = w_offset + w * stride;
// index of the data_col(reconstructed img)
int col_index = (c * height_col + h) * width_col + w;
data_col[col_index] = im2col_get_pixel(data_im, height, width, channels,
im_row, im_col, c_im, pad); // mapping pixel by pixel
}
}
}
}
im2col_cpu() accepts 2 pointer as input. Input pointer(a.k.a. image data pointer) points to start address of the image input, net.input + i*l.c*l.h*l.w
. Output pointer(a.k.a. col data pointer) points to the start address of the workspace, b = net.workspace
.
im2col_cpu() reconstruct image data $(c,h,w)$ to col data $(c\times size\times size, out_h\times out_w)$.
gemm() stands for General Matrix Multiplication. So weight matrix $(n, c\times size\times size)$ multiplies col data matrix $(c\times size\times size, out_h, out_w)$, finally get the output matrix $(n, out_h, out_w)$, for one single image. Note that pointer for *l.output has already points to the right place float *c = l.output + i*n*m
.
And for batch images, we will get the $(batch, n, out_h, out_w)$ output for *l.output.
Use add_bias() to add bias to *l.output and use activate_array() to pass through some chosen activation function. Conv forward done!
Just a review:
- Fill output with 0.0
- For each image:
- Convert input image data to col data
- Matrix multiplication between weights and col data to get the conv output(without adding the bias)
- Add biases to the output
- Pass through activation
Group Vision
Each image has been divided into l.groups groups, or more specifically, grouped by channels. So each group of image has the shape of $(\frac{c}{groups},h,w)$. Size of the filters is $[n\times \frac{c}{groups}\times size\times size]$. In other words, there are $l.n\times [\frac{c}{groups}\times size\times size]$ filters.
We don't use all the $l.n\times (\frac{c}{groups}, size, size)$ kernels to do conv operation with all $l.groups\times (\frac{c}{groups},h,w)$ partial-channel image, we group the filters as well as the image(actually image channels) first. Conv kernels have also been divided into l.groups groups, so each filter group has $\frac{l.n}{l.groups}\times (\frac{c}{groups},h,w)$ filters.
The image (channel) groups and filter groups are one-to-one correspondent. $Gropu_j$ filters are only responsible for $Group_j$ image, like, sort of conv pair.
int i, j;
int m = l.n/l.groups; // num of filters
int k = l.size*l.size*l.c/l.groups; // len of filter
int n = l.out_w*l.out_h; // len of output per output channel
for(i = 0; i < l.batch; ++i){
for(j = 0; j < l.groups; ++j){
float *a = l.weights + j*l.nweights/l.groups;
float *b = net.workspace;
float *c = l.output + (i*l.groups + j)*n*m;
// use im2col_cpu() to reconstruct input for each (input, weight) pair
im2col_cpu(net.input + (i*l.groups + j)*l.c/l.groups*l.h*l.w,
l.c/l.groups, l.h, l.w, l.size, l.stride, l.pad, b);
// conv operation(actually matrix multiplication) for one pair
gemm(0,0,m,n,k,1,a,k,b,n,1,c,n);
}
}
*a has the start address of $Group_j$ filters
*b has the start address of the workspace
*c has the start address of the output for $Group_j$ of $image_i$
net.input + (i*l.groups + j)*l.c/l.groups*l.h*l.w gives the address for $Group_j$ of $image_i$
Using im2col_cpu() each $(\frac{c}{groups},h,w)$ partial-channel image will get the 'partial-channel col data' of shape $(\frac{c}{groups}\times size\times size, out_h, out_w)$. Along with its $(\frac{n}{groups},\frac{c}{groups}\times size\times size)$ filters pair, do the matrix multiplication, outcome will be $(\frac{n}{groups},out_h,out_w)$.
Concatenating $groups \times (\frac{n}{groups},out_h,out_w)$ output, we will get $(n,out_h,out_w)$ output for one image as usual. The output shape remains the same, regardless of using this group thing.
Just a review:
- Image channels are divided into groups, so do the filters
- Image channel groups and filter groups are one-to-one correspondent, like conv pair. Do conv operation to each conv pair then concatenate to get the final output
- Num of the filter parameters $l.nweights=n\times \frac{c}{groups}\times size\times size$, reduced by a factor of l.groups
- Num of float operations become $\frac{n}{groups}\times \frac{c}{groups}\times size\times size\times out_h\times out_w\times groups$, reduced by a factor of l.groups
- Some global information may be lost, because conv operations do not cross the conv pairs.
E.G. No fuckin examples because it is stupid.
Binary things?
backward_convolutional_layer()
*l.delta has the same size of *l.output, as it will store the gradients w.r.t the output of current conv layer.
What forward_convolutional_layer() should compute for each input $x$ is $y=x\ast W$, and what it actually does is to compute $y=W\times x_{col}$.
So for backward_convolutional_layer(), it computes:
$\frac{\partial L}{\partial W}=\frac{\partial L}{\partial y}\cdot \frac{\partial y}{\partial W}=\frac{\partial L}{\partial y} \times {x_{col}}^T$
$\frac{\partial L}{\partial x_{col}}=\frac{\partial L}{\partial y}\cdot \frac{\partial y}{\partial x_{col}}=W^T\times \frac{\partial L}{\partial y}$
void backward_convolutional_layer(convolutional_layer l, network net)
{
int i, j;
int m = l.n;
int n = l.size*l.size*l.c;
int k = l.out_w*l.out_h;
// gradients pass through activation function
gradient_array(l.output, l.outputs*l.batch, l.activation, l.delta);
if(l.batch_normalize){
backward_batchnorm_layer(l, net);
} else {
backward_bias(l.bias_updates, l.delta, l.batch, l.n, k);
}
for(i = 0; i < l.batch; ++i){
for(j = 0; j < l.groups; ++j){
float *a = l.delta + (i*l.groups + j)*m*k;
float *b = net.workspace;
float *c = l.weight_updates + j*l.nweights/l.groups;
float *im = net.input+(i*l.groups + j)*l.c/l.groups*l.h*l.w;
im2col_cpu(im, l.c/l.groups, l.h, l.w,
l.size, l.stride, l.pad, b);
// compute gradients w.r.t. weights
gemm(0,1,m,n,k,1,a,k,b,k,1,c,n);
if(net.delta){ // if gradient descent continues(not the first layer)
a = l.weights + j*l.nweights/l.groups;
b = l.delta + (i*l.groups + j)*m*k;
c = net.workspace;
// compute gradients w.r.t. the reconstructed inputs(x_col)
gemm(1,0,n,k,m,1,a,n,b,k,0,c,k);
// reconstruct the im_col using col2im_cpu, resotre the struct,
// and get the gradients w.r.t. the inputs
col2im_cpu(net.workspace, l.c/l.groups, l.h, l.w, l.size, l.stride,
l.pad, net.delta + (i*l.groups + j)*l.c/l.groups*l.h*l.w);
}
}
}
}
$X$ has the shape of $(batch,c,h,w)$, and $X_{col}$ has the shape of $(batch, c\times size\times size, out_h, out_w)$.
For $Group_j$ in $Image_i$, $x_{col}^{ij}$ should have the shape of $(\frac{c}{groups}\times size\times size,out_h\times out_w)$.
$W$ has the shape of $(n,\frac{c}{groups},size,size)$. And what is responsible for $x_{col}^{ij}$, $w^{ij}$ has the shape of $(\frac{n}{groups},\frac{c}{groups}\times size\times size)$.
$\frac{\partial L}{\partial Y}$ has the shape of $(batch,n,out_h,out_w)$. What we need at a time is $\frac{\partial L}{\partial y^{ij}}$, has the shape of $(\frac{n}{groups},out_h\times out_w)$.
Given $\frac{\partial L}{\partial y^{ij}}$ and $x_{col}^{ij}$, call gemm(TA=0, TB=1, ...), we will get $\frac{\partial L}{\partial w^{ij}}=\frac{\partial L}{\partial y^{ij}}\times {x_{col}^{ij}}^T$, which has the shape of $(\frac{n}{groups}, \frac{c}{groups}\times size\times size)$. And will finally get the $\frac{\partial L}{\partial W}$, which will be stored in the memory block started from *l.weight_updates, of the size $(n,\frac{c}{groups},size,size)$.
Also, give $w^{ij}$ and $\frac{\partial L}{\partial y^{ij}}$, call gemm(TA=1, TB=0), we will get $\frac{\partial L}{\partial x_{col}^{ij}}={w^{ij}}^T\times \frac{\partial L}{\partial y^{ij}}$, which has the shape of $(\frac{c}{groups}\times size\times size, out_h\times out_w)$. And will finally get the $\frac{\partial L}{\partial X_{col}}$ of the shape $(batch, c\times size\times size, out_h, out_w)$, stored (start) from *net.workspace, $X_{col}$ will be overwritten.
Using col2im_cpu(), $\frac{\partial L}{\partial X_{col}}$ will be reconstruct to $\frac{\partial L}{\partial X}$, stored in the memory block that start from *net.delta, of the size $(batch,c,h,w)$.
Just a review (again...):
- Backprop through activation function
- Compute $\frac{\partial L}{\partial b}$, stored at l.bias_updates
- For each image(or each group/pair in each image):
- Compute $\frac{\partial L}{\partial W}$, stored at l.weight_updates
- Compute $\frac{\partial L}{\partial x_{col}}$, stored at net.workspace
- Use col2im_cpu() to reconstruct $\frac{\partial L}{\partial x_{col}}$ to $\frac{\partial L}{\partial x}$, stored at net.delta, namely the l.delta of the previous layer
update_convolutional_layer()
void update_convolutional_layer(convolutional_layer l, update_args a)
{
float learning_rate = a.learning_rate*l.learning_rate_scale;
float momentum = a.momentum;
float decay = a.decay;
int batch = a.batch;
axpy_cpu(l.n, learning_rate/batch, l.bias_updates, 1, l.biases, 1);
scal_cpu(l.n, momentum, l.bias_updates, 1);
if(l.scales){
axpy_cpu(l.n, learning_rate/batch, l.scale_updates, 1, l.scales, 1);
scal_cpu(l.n, momentum, l.scale_updates, 1);
}
axpy_cpu(l.nweights, -decay*batch, l.weights, 1, l.weight_updates, 1);
axpy_cpu(l.nweights, learning_rate/batch, l.weight_updates, 1, l.weights, 1);
scal_cpu(l.nweights, momentum, l.weight_updates, 1);
}
*l.weight_updates has the same size of *l.weights, *l.bias_update has the same size of *l.biases as well.